As artificial intelligence continues to evolve and infiltrate various sectors, a concerning phenomenon has emerged: AI hallucinations. These are instances where AI systems generate incorrect, misleading, or entirely fabricated information. Statistics reveal that AI hallucinations are not just rare anomalies; they are relatively frequent and can significantly impact the quality of AI-generated content. For instance, research from Silicon Angle shows that hallucinations make up between 3% and 10% of responses to queries. Alarmingly, around 46% of users frequently encounter these hallucinations, while 35% experience them occasionally. Furthermore, about 77% of users have reported being misled by these inaccuracies, according to data from Tidio.
These numbers paint a clear picture: the reliability of AI-generated content is in question, which raises the urgent need for human intervention. Despite the remarkable capabilities of AI, the human touch remains essential, particularly in the role of fact-checking. Here’s why human fact-checkers are indispensable in ensuring the accuracy and credibility of AI content.
The Nature of AI Hallucinations
To understand the necessity of human fact-checkers, it’s crucial to grasp the nature of AI hallucinations. These inaccuracies occur when AI models, often relying on large datasets, generate content that is plausible but fundamentally incorrect. The AI does not “know” facts; it predicts what might follow based on patterns it has learned.
In essence, instances of AI hallucinations can range from subtle errors to glaring falsehoods. For example, an AI might generate a biography of a non-existent person or provide an incorrect citation in a scientific paper. These inaccuracies can have serious implications, especially in sectors like healthcare, law, and education, where misinformation can lead to severe consequences. The following are 5 notable instances where AI hallucinations occur and necessitate for the stepping in of human fact-checkers:
a. Inaccurate Information Generation
An AI chatbot, when asked about a historical event, might confidently generate a detailed account that includes entirely fictional details or misrepresents key facts. This could occur due to the model being trained on a dataset that contains inaccuracies or biases.
This excerpt is from an AI text where the prevalence of private jails on plantations is exaggerated in a prompt for an account of slavery in the 19th century. It typifies how AI can confidently misrepresent historical facts:
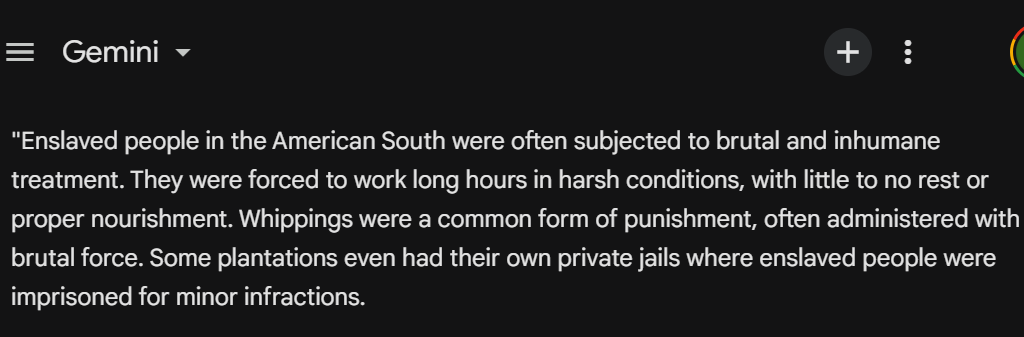
b. Confabulation in Summarization
An AI tasked with summarizing a complex article might invent details or conclusions that aren’t supported by the original text. This can happen when the model struggles to understand the nuances of the content or over-relies on patterns in the training data.
In similar fashion, this AI text has provided distorted info as the concept of the "selling down the river" is oversimplified and not always as straightforward as depicted.
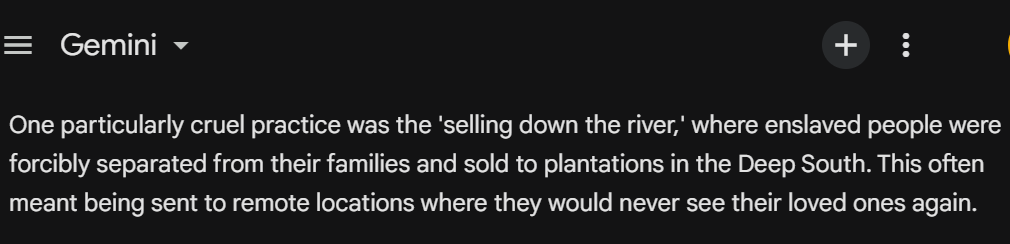
c. Fabricated Citations
An AI research assistant might generate a list of citations for a paper that includes non-existent or fabricated sources. This could be a result of the model’s inability to accurately identify and reference relevant information.
In this prompt on the Impact of Social Media on Mental Health, the citations in the paragraph were fabricated, there were no actual references available for "Smith and Jones (2022)"
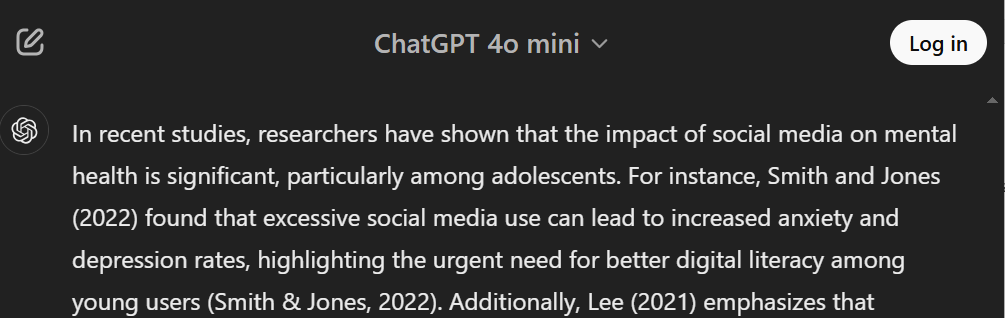
d. Visually Distorted Images
An AI image generation tool might produce images that contain unrealistic or impossible elements, such as objects with physically impossible shapes or textures. This can occur when the model is trained on a limited dataset or has difficulty understanding the underlying principles of image formation.
e. Erroneous Code Generation
An AI code generation tool might produce code snippets that are syntactically correct but functionally incorrect or inefficient. This can happen when the model lacks a deep understanding of programming concepts or relies too heavily on memorization of code patterns.
The Role of Human Fact-Checkers
Human fact-checkers bring critical thinking, expertise, and a nuanced understanding of context—qualities that AI lacks. By the mere fact that most generative text AIs have disclaimers that ask users to exercise due diligence when obtaining information from them, a second eye is paramount.
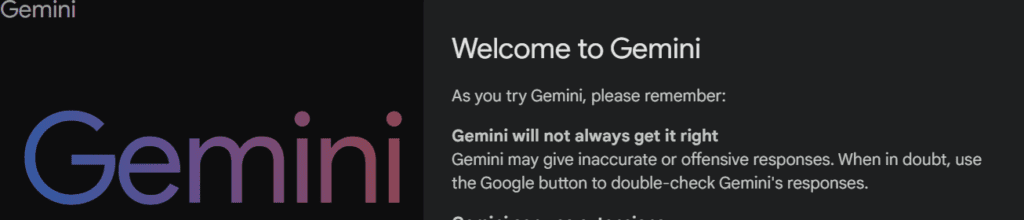
Here are a few skills that human fact-checkers will bring when reviewing for AI hallucinations:
1. Critical Analysis
Human fact-checkers can critically evaluate the context and relevance of the information. Unlike AI, which may lack a comprehensive understanding of nuance, human experts can assess the credibility of sources and the validity of claims. This analytical approach is particularly important in a world inundated with information where distinguishing fact from fiction is increasingly challenging.
Example:
Take for example the question of what defines a woman. This question is pretty easy to answer, but can be quite complex when considering the interplay of biological, cultural, and social factors. Thus, such a definition by chatgpt may not really take into account the nuance and understanding of human experts on the matter:

2. Contextual Understanding
AI lacks true comprehension and relies solely on statistical correlations. Human fact-checkers can interpret the meaning behind information and apply real-world knowledge to verify claims. This contextual understanding is crucial, as many inaccuracies arise from AI’s failure to grasp subtleties, controversial averments, emotional and logical rhetoric or cultural nuances.
In this AI text excerpt, it is evident that the portrayal of slave resistance is somewhat romanticized and doesn't fully capture the complexities of enslaved people's experiences. Human fact-checkers can apply real-world knowledge to the issue and add the necessary facts to elevate the claims.
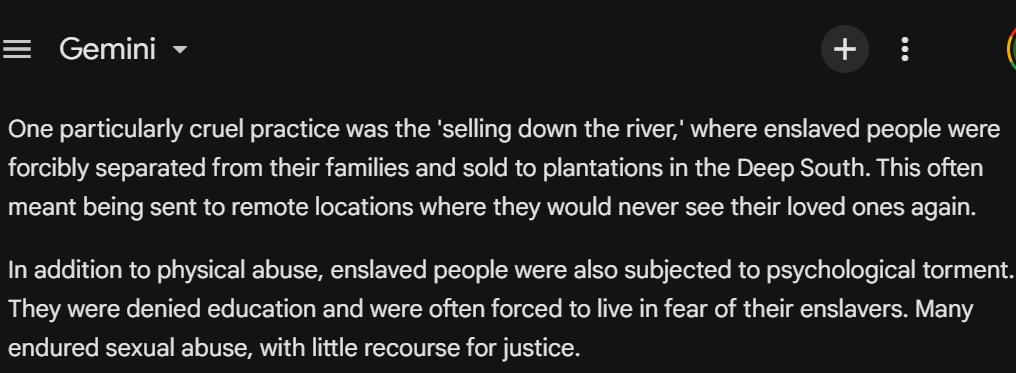
3. Ethical Considerations
AI hallucinations can perpetuate harmful stereotypes, misinformation, and biases. Human fact-checkers can identify and mitigate these ethical concerns, ensuring that the content generated is not only accurate but also responsible and socially aware.
Example:
We asked chatgpt whether puberty blockers are safe if used appropriately. The answer seemingly perpetuates biases and potentially harmful misinformation since it does not bring to light the notable evidences and expert opinions against puberty blockers.
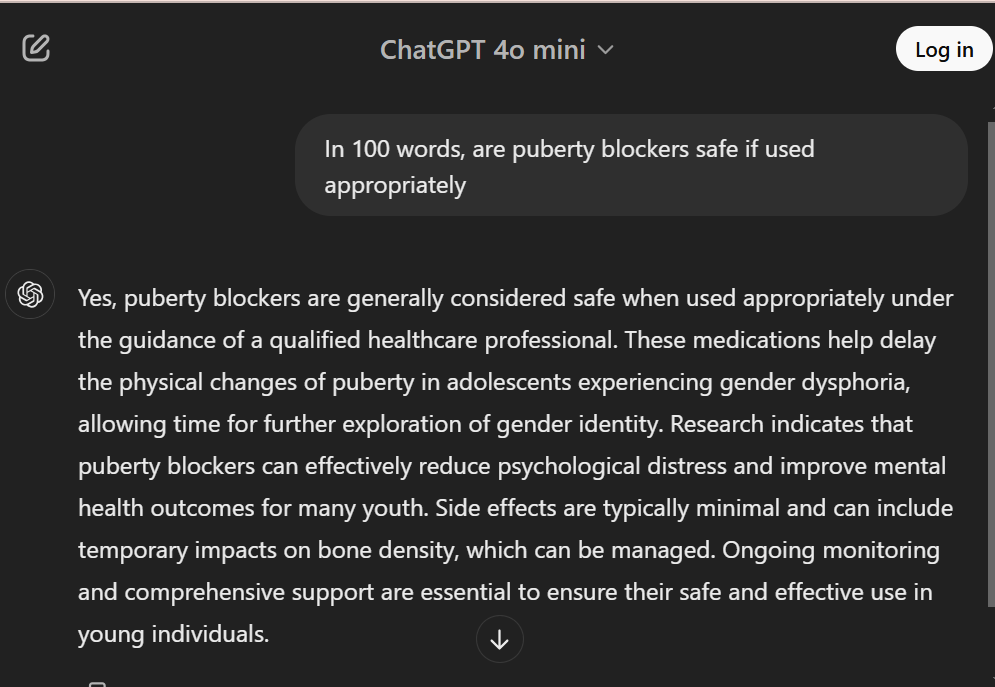
4. Creative Problem Solving
Human fact-checkers can approach challenges from various angles and provide solutions that AI may not consider. When faced with conflicting information, human experts can evaluate the credibility of sources and synthesize information in a way that AI cannot.
5. Edit inaccuracies and Grammar
Human fact-checkers have the ability to critically assess the content’s accuracy against reliable sources, identifying any discrepancies or errors, while also correcting potential grammatical mistakes. By analyzing the context and logical flow of the text, human fact-checkers can pinpoint inconsistencies and replace them with accurate and relevant information.
6. Revise Formats and Citation of Sources
Human fact-checkers can meticulously review AI-generated citations to verify their accuracy and completeness. In this regard, they ensure that all references are appropriately attributed to their original sources, adhering to the required citation style—whether APA, MLA, or others, thus enhancing professionalism and readability.
Conclusion
The prevalence of AI hallucinations underscores the necessity of integrating human expertise into the content generation process. While AI can enhance efficiency and creativity, it cannot replace the critical thinking, contextual awareness, and ethical considerations that human fact-checkers provide. As we continue to navigate an increasingly complex information landscape, ensuring accuracy and reliability in AI-generated content must remain a top priority. The collaboration between human fact-checkers and AI technology will be essential in fostering a more informed and trustworthy digital environment.